How to choose an attribution model, advice from a data scientist
The data-driven way to choose an attribution model

First Touch,
Last Touch,
Last Non-Direct Click,
Time Decay,
Linear,
Any Touch š,
Last Paid Touch,
U-shaped,
W-shaped,
Algorithmicā¦.
Iāve spent many years on the data-side of marketing teams. One thing Iāve learned is that so many companies fall into the same trap when choosing an attribution model.
Every company wants to be data-driven so they assume they need that fancy multi-touch attribution model to capture all the complex nuances of their customerās behavior and what led them to purchase.
ā¦and this is exactly what the $130B/yr ad-tech industry wants us to think.
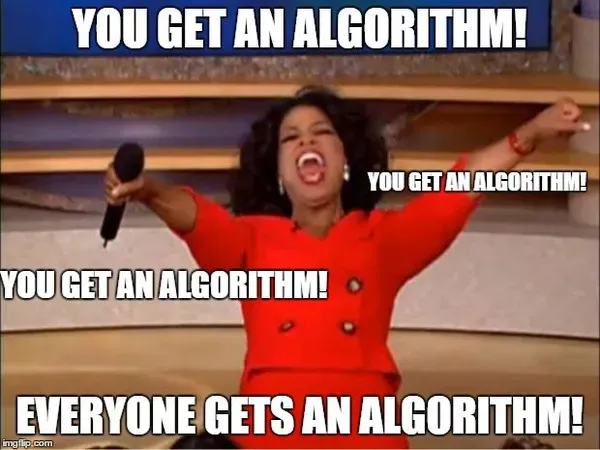
After seeing these same patterns play out at large enterprises like IBM, unicorn startups like WeWork, and tons of small startups⦠Iāve come to realize one thing:
Fancy multi-touch attribution models are overhyped. Most likely, you donāt need one. There, I said it.
The truth is, Iāve looked at the data so I can objectively say that the vast majority of companies do not need a fancy multi-touch model (especially if youāre an e-commerce / D2C brand).
Even worse, when we opt for a fancy multi-touch algorithmic model unwarranted, weāre inviting a whole slew of problems that could have been avoided entirely if we had just taken the time to choose an appropriate attribution model up front.
The downside of complex attribution models
An overly-complex model can mean a lag in reporting while you wait for customers to convertāāāor alternatively, youāll have metrics that retroactively change as more data is acquired. Of course, thereās the added maintenance for your data team and the lost budget on said fancy-attribution product you didnāt need.
Finally, letās not forget the added confusion for the marketing practitioners who need to act on the results.
All of this can be avoided by taking the time to look at the data, understand what your customers are doing, and choose an appropriate attribution model up front.
Ok, so let's talk about choosing an attribution model...
DOs and DONāTs of Choosing an Attribution Model
DONāT
- Choose a model based on the specific campaign that youāre evaluating
Ex. upper funnel campaigns get first-touch attribution. This is cheating. - Rely on the models used by your Ad Platforms
First off they donāt have access to all of the data they need to properly attribute conversions.
Secondly, theyāre notoriously bad at math and their āmistakesā usually make their platform seem more favorable (see Facebook in 2016, 2017, 2018, 2020 š). - Implement a handful of models and see which āmakes the most senseā
This is a big headache and often leads to bias and conditional attribution (see point #1).
DO š
- Choose your attribution model objectively, with data!
This is data-driven marketing. Weāll get into the approach in a sec. - Use the data in your data warehouse so you can use the source-of-truth for conversion activity (not pixels) and combine data from all your ad sources
In order to understand things like ROI, you need the full picture. How much was spent across ALL of your ad sources to acquire that customer?
How to choose your attribution model, with data!
At the end of the day, a good attribution model is objective and universally applied across all ad sources. And our goal is to choose the model that minimizes complexity while capturing the nuances of each customerās unique behavior.
To do this we need to understand how customers are behaving, so we start by looking at the data and systematically run through a series of checks that guide us to the right answer.
Hereās the approach we use at Narrator to decide on an attribution model:
1. Do most customers convert on their first visit?
If YES ā Use a First Touch model.
Easy choice. Thereās no question about how to assign credit when customers only have one session before converting. By the way, this behavior is pretty common. Weāve done this analysis for many companies and youād be surprised by how many of them fall into this group (especially e-commerce brands).
If NO ā We need more info to choose a model, move to the next question.
We need to understand if thereās a media mix before we can choose a model.
2. Do customers usually engage with different ad sources before converting? (Is there a media mix?)
If YES (not common) ā Use a multi-touch model.
Since customers are engaging with multiple ad sources, we need to use a model that distributes credit across each source. Weāll need a multi-touch model.
There are many different kinds of multi-touch models and the choice of model is a tradeoff between resources/complexity vs accuracy. The most straight-forward multi-touch approach is a linear model, but if you have the resources to implement a more advanced model we recommend using a logistic regression approach to assign partial credit.
If NO ā We can use a single-touch model, the next question will help us determine which one.
We donāt need to distribute credit amongst the paid touches because there isnāt a media mix. This means we can use a single-touch approach (last touch/first touch/last-paid touch).
3. Do customers who initially visit via organic, come back via a paid source?
If YES ā Use a Last Touch or Last Paid Touch model.
If we were to use a first-touch model we'd be masking the contribution of paid touches later on in the customer's journey. This is why we'll need a last touch or last-paid touch model to assign credit accordingly. Use last-paid touch if you'd like to ensure credit is always given to paid marketing.
If NO ā Use a First Touch model.
Since organic visitors won't come back via paid sources, we can confidently use a first-touch model without the risk of masking credit from subsequent paid touches. Essentially, when people have a paid touch in their journey, it's the first touch. That's why we can use a first-touch model.

Attribution models in the wild
At Narrator, weāve had the opportunity to battle-test the approach with many different companies: subscription, e-commerce, direct to consumer, B2B, retail, etc. Every time, itās yielded some surprising insights.
Mostly, because we all assume that our customers are re-visiting the site multiple times and engaging with lots of different ads along the way. But when we look at the data, we learn that this behavior very unusual.
And, with any analysis, it's important to re-check these questions periodically to see if your customers' behaviors have changed. This will ensure you're always using the right attribution model. At Narrator, we use Narratives to automatically re-run our analyses on a regular basis and monitor changes in customer behavior (ex. we run our attribution analysis weekly just to be safe).
If your team is considering different attribution models, I highly recommend looking at your data to answer these questions before getting started. It may help you save your time and resources for the more important questions that actually require those fancy data science models š